Key Points:
- Drugs that extend the lifespan of mice, such as rapamycin, have already been discovered, but AI can help identify more potent drugs.
- Several longevity drugs discovered by AI have already made it to clinical trials.
- AI could potentially be used to generate drugs that target all 12 purported underlying causes of aging.
“When you’re baby steps away from having the powers of the gods, in this case, artificial intelligence, the only foe is death.” — Bryan Johnson
At the turn of the millennium, aging research went into overdrive as scientists began tinkering with the lifespans of yeast, worms, and flies. In turn, they discovered how to modulate the aging process by targeting the same cellular signaling pathways shared with humans.
Eventually, in 2014, a milestone was reached when the drug rapamycin was demonstrated to extend the lifespan of both male and female mice by targeting the mTOR signaling pathway. Since then, researchers have identified a myriad of drugs and supplements capable of extending the lifespan of various animal models.
Now, many individuals take these anti-aging pills in the hope of prolonging their lifespan. For instance, Bryan Johnson, whose slogan is “DON’T DIE, supplements with over 40 anti-aging pills every day. Johnson’s supplement stack includes rapamycin, which is still the most potent anti-aging drug to date.
However, one of the foremost experts on rapamycin David M. Sabatini, M.D., Ph.D., said on The Peter Attia Drive Podcast that he thinks there are more targets in [the mTOR signaling] pathway waiting to be discovered. These targets may be more beneficial than rapamycin’s target — mTOR.
In many ways, Dr. Sabatini’s sentiment reflects the current landscape of aging research: there are more important discoveries to be made. For this reason, many scientists have looked towards the capabilities of artificial intelligence (AI).
AI Saves Time and Resources
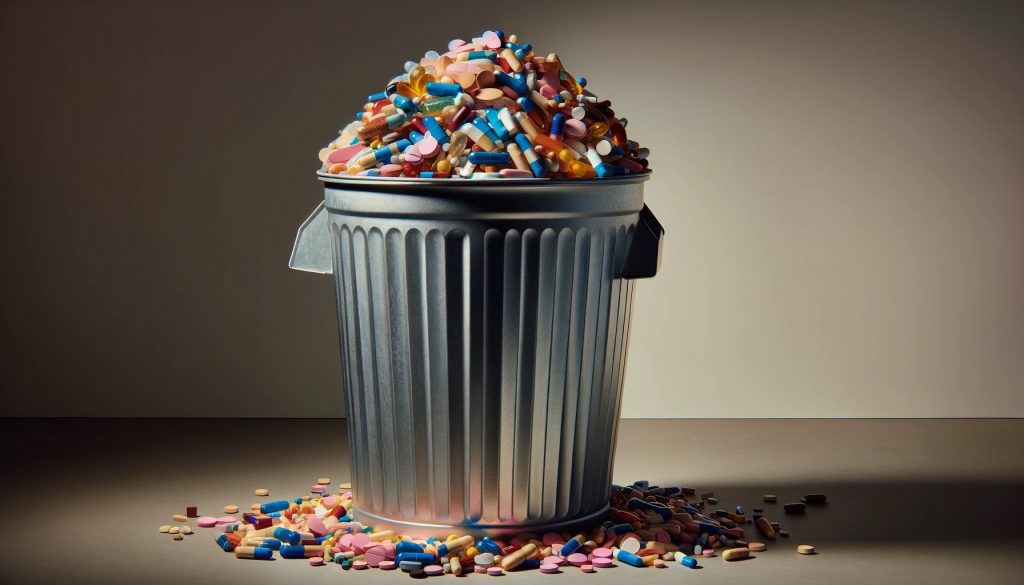
The beauty of rapamycin is that when researchers first used it to extend the lifespan of mice it was already FDA-approved. Because of this, much of the costs, risks, and time associated with finding a new drug were avoided. This is critical because new drugs typically take around 10 years and about $2 billion to bring to market.
Moreover, less than 10% of new drugs successfully transition from R&D to the clinic, generating ample throw-away drugs. Such failures account for the majority of drug development costs.
To limit the wasting of time and resources, many researchers have begun utilizing AI-driven drug discovery. Scientists can use AI to identify new targets and signaling pathways that underlie diseases like cancer, neurodegenerative disorders, and autoimmune conditions. In turn, AI systems like AlphaFold can predict the 3D structures of these targets (proteins) to determine which drugs they will bind.
If that wasn’t enough, some AI systems can even come up with entirely new drugs from scratch. By discovering new targets and generating new drugs, AI can crunch the drug discovery timeline to 18 months.
AI Discovered Anti-Aging Drugs
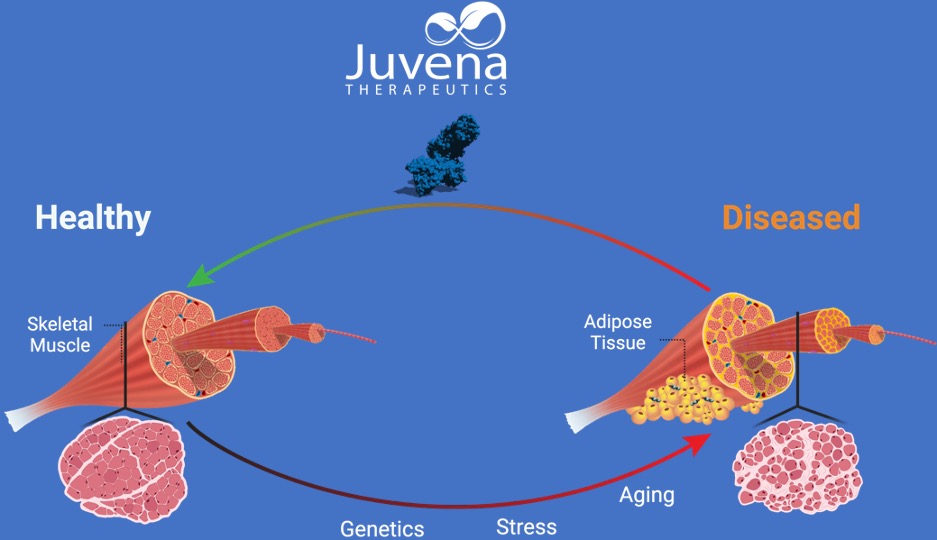
AI’s drug discovering capabilities have already been demonstrated in the real world. Most recently, Insilco Medicine reported its phase I clinical trial results, showing the safety and tolerability of an AI-discovered drug designed to treat idiopathic pulmonary fibrosis, an age-related lung scarring disease. Also making it to clinical trials is Juvena Therapeutics’ AI-discovered drug JUV-161, which may help to slow muscle aging.
Furthermore, Kaempferol, which has been shown to slow the progression of Alzheimer’s disease in animal models, was identified by AI technology and in a recent clinical trial revealed to be safe and tolerable in healthy adults. However, Kaempferol has yet to be tested in patients with Alzheimer’s. Based on these and other studies, AI-driven drug discovery may become the standard when it comes to identifying new therapeutics.
Curing Aging with AI-Discovered Drugs
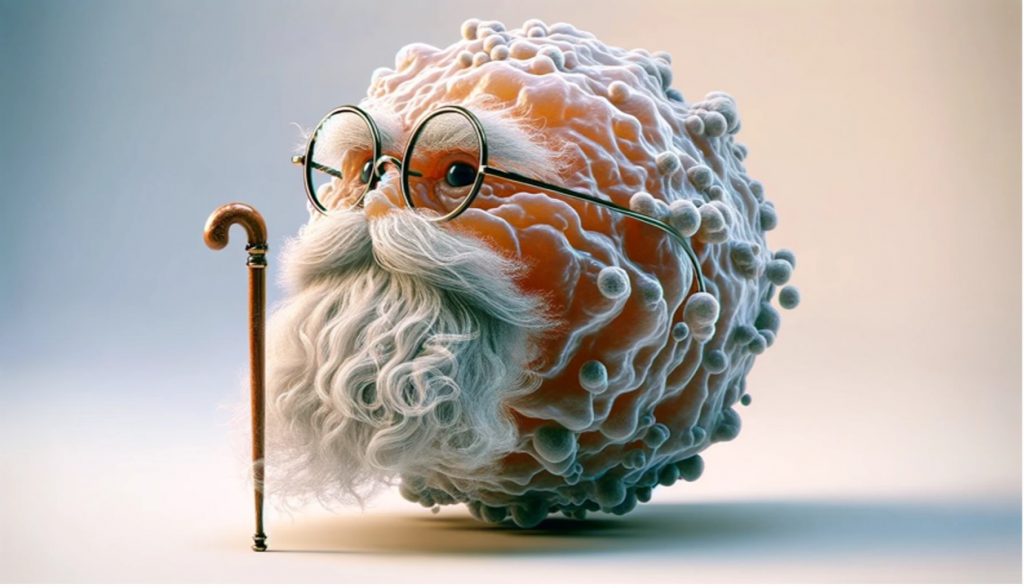
The primary risk factor for many chronic diseases, including neurodegenerative disease, heart disease, and cancer is aging, making these chronic diseases age-related. Remarkably, over the past few decades, scientists have uncovered 12 cellular defects that underlie nearly all age-related diseases. It follows that if these cellular defects, called the hallmarks of aging, could be treated simultaneously, then, hypothetically, aging could be cured.
The problem is that scientists have yet to elucidate the complex interplay between the multiple signaling pathways involved in the hallmarks of aging. However, considering exponential technological advancements, such a problem may be easy to solve with AI, as long as it’s given the right data. Therefore, it is possible that AI could be harnessed to identify combinations of drugs that target all 12 hallmarks to halt the progression of aging.
So far, things appear to be on the right track, as MIT and Harvard researchers have utilized AI to identify a drug shown to reduce senescent cells, one of the hallmarks of aging. Moreover, this drug, a senolytic, outperformed at least one existing senolytic drug. At this rate, we may eventually have the perfect drug for each hallmark of aging. Still, the true test will be whether any of these drugs slow the progression of aging in humans.